Developed by the Artificial Intelligence Division in Simulation, Education, & Training at University of Arizona Health Sciences, Artificially Intelligent Medical History Evaluation Instrument (AIMHEI) uses artificial intelligence to enhance medical education by providing consistent, personalized feedback in history-taking and clinical skills. This innovative AI tool offers medical students real-time assessments focused on medical terminology, empathy, and communication. Its aim is to empower students to refine clinical reasoning and patient interaction skills effectively, without overburdening faculty.
Imagine you are a young medical student, standing in front of a patient for the first time. You need to take a full medical history, ask the right questions, listen carefully, and respond with empathy. It is a crucial skill that requires constant practice and targeted feedback. But what if your mentors are not always available, or their evaluations vary widely? At a time when technology is transforming fields such as finance and industry, why can’t it do the same for medical education? This is where artificial intelligence steps in. The Artificially Intelligent Medical History Evaluation Instrument (AIMHEI), an advanced AI system, promises to provide immediate, personalized feedback to future doctors, bridging a critical gap in clinical training.
The Context
Medical education continues to face challenges in providing students with frequent and personalized feedback on essential skills like taking medical history and oral presentations. While faculty mentors are crucial in guiding student development, their availability is often limited, and students frequently seek additional opportunities to practice essential skills (Case et al,2024). To address this gap, we developed the Artificially Intelligent Medical History Evaluation Instrument (AIMHEI), an AI-powered tool designed to give real-time, individualized feedback to medical students. This tool aims to support students in mastering communication and clinical reasoning while reducing the burden on faculty.
These skills, foundational to clinical practice, are defined as core entrustable professional activities (EPAs) for residency outlined by the Association of American Medical Colleges (AAMC, 2014). However, many residency program directors have expressed concerns that students may not fully develop their communication skills and medical knowledge before intern year (Tischendorf et al, 2018; , Lyss-Lerman et al, 2019) This is largely due to lack of frequent and structured feedback during training, stemming from limited faculty availability and demands of patient care. Furthermore, feedback provided by preceptors or attending physicians can be subjective, vary across specialties, and is often time-consuming to deliver (Barrows, 1993; Miller, 1990)).,
These challenges limit students’ ability to refine these skills and implement feedback.
How Artificial Intelligence can help
Artificial intelligence (AI) offers a promising solution to these challenges by providing scalable, on-demand feedback. Artificial intelligence has demonstrated many promising applications in medical education, particularly as a tool to help medical students engage with complex clinical topics in real-time (Rosipigliosi, 2023) ChatGPT, for instance, has shown potential in tracking students’ progress and adjusting its teaching style to match their individual learning needs (Lee, 2023; Chan& Zary, 2019)., Notably, ChatGPT has successfully passed the United States Medical Licensing Examination Step 1 based on practice questions, providing clear and comprehensible explanations. AI has repeatedly demonstrated its capacity to serve as an interactive, on-demand resource, offering significant value for refining medical knowledge (Kung, 2023).
The AIMHEI will not only provide medical students with real-time, individualized feedback on these core competencies but can also ensure the quality and consistency of the feedback, thereby helping to refine them. This allows medical students to practice their communication and clinical reasoning skills necessary for real-life clinical situations without overburdening faculty resources.
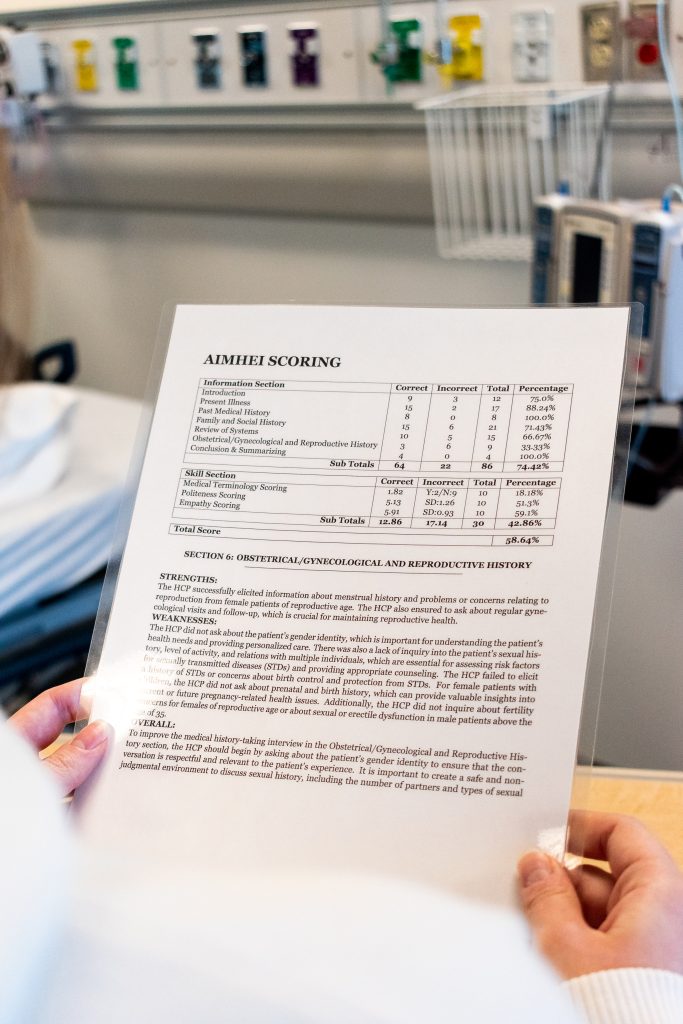
AIMHEI Functionality and Key Features
The program is a culmination of Python scripts and Natural Language Processing (NLP) methodologies. It consists of two major components that are used to score medical students: an information and skills section. The information section contains a checklist type rubric that measures endpoints derived from Bates’ Guide to Physical Examination and History Taking. (Bickley & Szilagyi, 2016). The information section criteria are contingent upon the specific learning objectives and desires of the educators and/or overseers. The skills section consists of Medical Terminology Scoring, Politeness Scoring, and Empathy Scoring, all of which are designed to address specific aspects of a medical interview and provide coaching feedback for improvement and recognition of successes.
In practice, AIMHEI is used to analyze a transcript generated from an audio recording of an interview with a standardized patient. The transcript is reviewed and scored alongside 96 different criteria. AIMHEI produces two reports from this score, a faculty report and a student report. The faculty report contains a line-by-line justification of the score, including what the criteria was, an explanation for the given score, an in-line citation from the transcript providing evidence for the score, and the section the criteria fall into. It is extremely thorough and equipped to justify itself should a faculty or student need to traceback a score.
This faculty report is then converted into a student report within seconds. The student report is provided to the student shortly after completing the interview. This AIMHEI report, contains an abridged version of the faculty report while still providing the pertinent information, including a breakdown of each section and subsection with performance scores. At the end of the report, customized feedback is produced for each transcript the program reviews. The customized feedback is derived from low scoring sections for the student to focus on improving for the next visit. The diagram below shows the workflow of the AIMHEI program from start to finish.
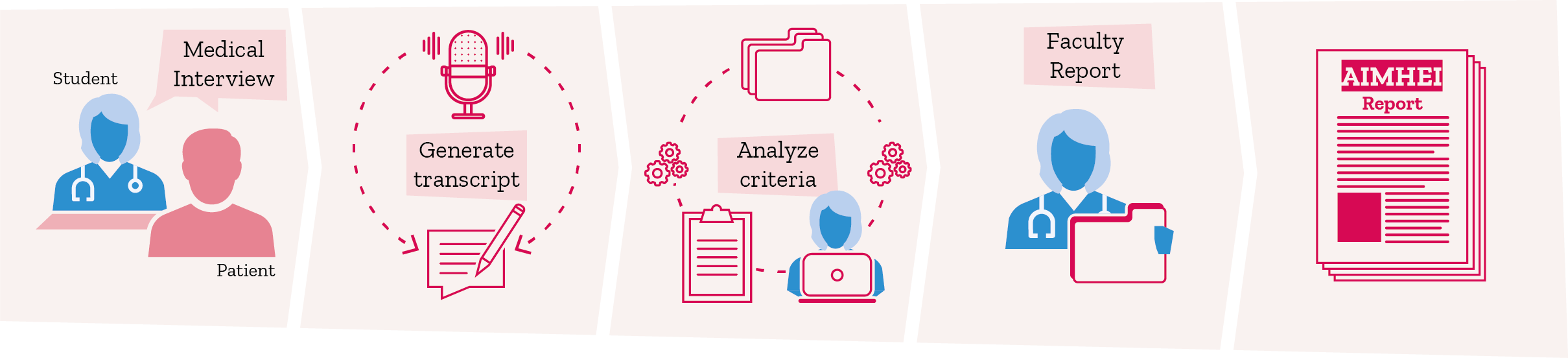
What is next?
Currently, we are conducting a randomized controlled trial (RCT) to evaluate the effectiveness of the AIMHEI among medical students. In this trial, we are collecting feedback from students on the quality and usefulness of the AI-generated assessments, as well as tracking their progress in developing medical history-taking skills over the course of their first year. This builds on a successful pilot study, where we gathered initial feedback on the organization and clarity of the feedback provided by AIMHEI. The results from the pilot allowed us to make improvements to the tool’s feedback system, ensuring that it is structured and actionable for students.
Looking beyond the current trial, AIMHEI has the potential for broader applications in medical education. One future direction involves adapting the tool to provide feedback on other core competencies, such as the oral case presentation, which is critical for both clinical reasoning and effective communication. Additionally, AIMHEI could be refined for specialty-specific feedback, allowing it to cater to the unique communication and clinical skills required in fields such as surgery, internal medicine, or pediatrics. By expanding the tool’s capabilities, AIMHEI can further support medical students as they develop the diverse skill sets needed for their chosen specialties, while also offering a scalable solution for faculty to manage student feedback across different domains.
Last thoughts
The development of this language processing program marks a significant advancement in medical education technology. By focusing on the key aspects of medical interviews – medical terminology accuracy, politeness, and empathy – the program promises to enhance the quality of future medical professionals’ patient interactions. As this technology integrates further into medical education, it holds the potential to transform how medical professionals communicate, ultimately leading to improved patient care and satisfaction.
Bibliography
Case, L., Khan, I., & Qato, K. (2024). The past, present, and future of feedback in medical education. Journal of Vascular Surgery- Vascular Insights, 2, e100116. https://doi.org/10.1016/j.jvsvi.2024.100116
Drafting Panel for Core Entrustable Professional Activities for Entering Residency. (2014). Core Entrustable Professional Activities for Entering Residency: Faculty and Learner’s Guide. Association of American Medical Colleges. https://store.aamc.org/downloadable/download/sample/sample_id/66/%20
Tischendorf, J., O’Connor, C., Alvarez, M., & Johnson, S. (2018). Mock Paging and Consult Curriculum to Prepare Fourth-Year Medical Students for Medical Internship. MedEdPORTAL: The Journal of Teaching and Learning Resources, 14, e10809. https://doi.org/10.15766/mep_2374-8265.10708
Lyss-Lerman, P., Teherani A., Aagaard, E., Loeser, H., Cooke, M., Harper, G. M. (2009). What training is needed in the fourth year of medical school? Views of residency program directors. Academic Medicine: The Journal of the Association of American Medical Colleges, 84(7), 823-829. https://doi.org/10.1097/ACM.0b013e3181a82426
Barrows, H. S. (1993). An overview of the uses of standardized patients for teaching and evaluating clinical skills. Academic Medicine: Journal of the Association of American Medical Colleges, 68(6), 443–453. https://doi.org/10.1097/00001888-199306000-00002
Miller, G. E. (1990). The assessment of clinical skills/competence/performance. Academic Medicine: Journal of the Association of American Medical Colleges, 65(9 Suppl), S63-S67. https://doi.org/10.1097/00001888-199009000-00045
Rosipigliosi, P. A. (2023). Artificial intelligence in teaching and learning: What questions should we ask of ChatGPT? Interactive Learning Environments, 31(1), 1-3, https://doi.org/10.1080/10494820.2023.2180191
Lee, H. (2023). The rise of ChatGPT: Exploring its potential in medical education. Anatomical Sciences Education, 17(5), 926-931, https://doi.org/10.1002/ase.2270
Chan, K.S. & Zary, N. (2019). Applications and Challenges of Implementing Artificial Intelligence in Medical Education: Integrative Review. JMIR Medical Education, 5(1), e13930. https://doi.org/10.2196/13930
Kung, T.H., Cheatham, M., Medenilla, A., Sillos, C., De Leon, L., Elepaño, C., Madriaga, M., Aggabao, R., Diaz-Candido, G., Maningo, J., & Tseng, V. (2023). Performance of ChatGPT on USMLE: Potential for AI-assisted medical education using large language models. PLOS Digital Health, 2(2), e0000198. https://doi.org/10.1371/journal.pdig.0000198
Bickley, L. S., & Szilagyi, P. G. (2016). Bates’ Guide to Physical Examination and History Taking (12th ed.). Lippincott, Williams & Wilkins.ommunication and empathy skills. BodyInteract Blog. 2023. https://bodyinteract.com/blog/communication-empathy-virtual-patients/
READ ALSO