Artificial Intelligence in healthcare simulation, once seen as science fiction, is reshaping medical training. From virtual patient simulators to AI-guided nursing and surgical modules, today’s technology delivers learning experiences that evolve in real time—something only the most skilled instructors could have achieved before. When a student interacts with a virtual patient, AI processes thousands of data points, from vocal tone to procedural precision, adapting the scenario on the fly. This new frontier in medical simulation is bridging theory and practice, creating healthcare professionals who are not only technically adept but also adaptable and compassionate.
Imagine a medical student practicing a complicated procedure: instead of the usual manikin, she works with an Artificial Intelligence (AI)-powered virtual patient who responds in real time, provides immediate feedback, and even simulates complications. It’s not science fiction, it’s happening in medical schools today and is changing how we train health professionals.
The Evolution of Medical Simulation:
The journey from basic manikins to AI-powered simulation probably represents a paradigm shift in medical education. Traditional means of simulation had clear limitations. Standardized patients are only able to simulate a finite number of conditions; manikins do not possess the subtle physiological changes that are very important for learning, and students often have to imagine critical clinical signs rather than observe them directly. Enter artificial intelligence, and suddenly these limitations start to dissolve. Using contemporary simulators based on AI, it is possible to recreate a complex situation of a patient that demonstrates subtle physiological changes and even reacts autonomously to what the student is doing. It’s a technological advance that is not about better graphics or using ever-more-realistic manikins but about creating truly interactive learning experiences that adapt and respond to the needs of each student.
Inside the AI Engine: How It Actually Works
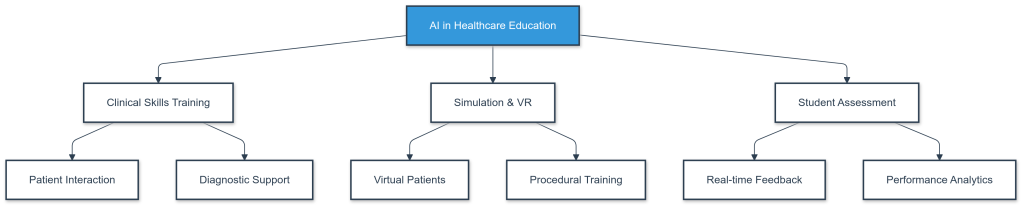
AI stands apart from traditional computing methods due to its ability to emulate human-like thinking and problem-solving. As Prof. Hamilton A. explains well in a recent article on AI and healthcare simulation (2024), in a nutshell, AI differs from computer systems of the past for four main reasons: (1) it learns from data and adapts its decisions based on that learning; (2) it can access vast databases, sometimes representing a significant portion of online knowledge; (3) it handles diverse tasks without specific programming; and (4) it can generate code independently to complete tasks when needed.
AI replicates human intelligence within machines, enabling them to perform functions such as reasoning, problem-solving, learning, perception, and decision-making. It encompasses a diverse array of methods and applications, including natural language processing, machine learning, and computer vision. Let’s examine them in detail with some examples.
Natural Language Processing
Natural Language Processing (NLP) is a branch of AI focused on enabling computer systems to analyze, interpret, and understand human language in both spoken and written forms. NLP processes text or voice inputs to tackle different elements of language, such as syntax (the structural arrangement of words and phrases) and semantics (the conveyed meaning). To achieve this, NLP leverages a blend of techniques, including rule-based methods, statistical approaches, machine learning, and deep learning models, which together allow it to interpret language and generate meaningful responses. (Reading Turchioe M, et al., 2022)
Furlan R and colleagues (2021) developed a virtual patient simulator named Hepius that, by natural language interaction and intelligent tutoring systems, helped students to improve their clinical diagnostic reasoning skills without necessarily requiring the presence of human tutors or the need for the student to be at the bedside of a real patient. Interaction in natural language between the student and the program was created using three distinct programming languages to facilitate the tasks of anamnesis, physical examinations, medical test ordering, and generating diagnostic hypotheses. Additionally, the authors shared preliminary results from a short-term learning assessment conducted on undergraduate students after engaging with the simulator.
Machine Learning
Machine Learning (ML) algorithms are capable of “learning” from data without being explicitly programmed, and can solve a wide range of problems, including face recognition, machine translation, creative text writing and medical diagnosis. In the area of healthcare simulation, the case presented by a group of researchers from Queen’s University in Kingston, Ontario, Canada, who developed an augmented reality (AR) adaptive simulation platform for medical training is interesting. (Ruberto et al., 2021) The main goal was to create a more effective training tool that, using AI algorithms, could adapt to the different skill levels and stress responses of participants, making the learning experience more efficient and beneficial. The platform was designed to dynamically adapt to the cognitive load of medical students and physicians-in-training. Participants wore Augmented Reality headsets, which superimposed digital images of a patient onto a physical mannequin. This created a realistic and interactive training environment. At the same time, the system measured participants’ cognitive load by tracking heart rate variability and galvanic skin response in real time through sensors. The simulations, then, evolved according to participants’ stress levels and cognitive abilities: if a participant showed signs of high cognitive load, indicating stress or overwhelm, the simulation reduced the severity of the patient’s symptoms to better manage the learning experience.
In another study conducted by Belmar and colleagues (2024), the results showed that developing AI algorithms to evaluate fundamental laparoscopic simulation training exercises is not only feasible but improve assessment accuracy: the findings, in fact, revealed that the application of AI can have high levels of agreement with the expert reviewers, who are currently considered the gold standard in this field.
In a 2012 study conducted at the University of North Carolina at Chapel Hill, a fusion of technology and surgical skill assessment was conducted. (Watson RA, 2014) Fourteen experienced surgeons and 10 residents performed two vascular surgery (venous anastomosis) simulations. Their hand movements were tracked with a device, transforming their actions into symbolic time series. This data was then used to train a machine learning model, known as Support Vector Machine, to distinguish between expert and novice movements. The model achieved 83 percent accuracy in identifying skill levels. In addition, an algorithm used for data compression, applied to hand movement patterns, blindly classified hand movement patterns into expert and novice groups with 70% accuracy, offering a new approach to objectively assessing surgical competence.
Computer vision technology
Imagine giving computers the ability to “see”! Computer vision allows machines to extract meaningful information from digital visuals, performing tasks like image recognition, object detection, image segmentation, and even creating new images.
Islam and Kahol (2011) proposed a video-based approach for observing surgeon’s hand and surgical tool movements in both surgical operation and training. The video-based method recorded continuous sequences of surgeons’ hand, posture, and tool movements throughout entire surgical procedures using a low-cost video camera. The video data was analyzed with a computer vision algorithm and correlated with each surgeon’s skill level. To model surgical skills, a stochastic approach processed the data, and a data mining technique developed an observer-independent model based on objective, quantitative skill measurements. This non-contact tracking system minimally interfered with the performance of operations as well as avoiding the sterility problems.
But according to Dr. Martinez from the Eastern Health Institute, the real magic is made possible with the integration of the three technologies: Natural Language Processing enables virtual patients to engage in realistic conversation, understanding not just what students say but how they say it. Continuing analysis by machine learning algorithms, with subtle changes in scenarios, provide just the right degree of challenge. Perhaps most impressively, computer vision technology watches and analyzes student movements during procedures, now providing guidance that formerly required constant faculty supervision. «What amazes me» says Dr. Martinez, «is how these technologies work together seamlessly. When a student performs a procedure, the system isn’t just checking off boxes. It’s understanding the whole interaction — the technique, the communication, the decision-making — just like an experienced instructor would».
When Virtual Meets Reality: Some Success Stories
Medical training is seeing a real transformation with the use of virtual and augmented reality (VR and AR), especially in surgical education. These tools create immersive, 3D environments where medical students and residents can practice complex skills in ways that feel close to real life. Studies have shown that VR-trained students can complete procedures much faster and with greater accuracy than those using traditional methods—sometimes up to 43% faster. This isn’t just a time-saver; it also makes training more affordable, as VR systems reduce the need for costly, real-life practice setups.
Imperial College London has integrated virtual reality (VR) into its medical training to prepare students for emergency situations like cardiac arrest and severe asthma attacks. These VR simulations give students a realistic, hands-on experience where they can make critical decisions without the pressure of real-life consequences. According to Dr. Risheka Walls, this approach enables students to develop confidence and practical skills in a “safe space” setting. Fifth-year student Thivyaa Gangatharan highlighted how VR helped her move from theoretical learning to practical action, creating lasting memories of emergency procedures. The program’s success has gained wide attention and is set to expand with support from NHS England.
Human Touch in Digital Training
One of the most inspiring aspects of AI in medical simulation is its role in enhancing, not replacing, human connections in healthcare training. For example, AI-powered virtual patient simulations offer students a safe space to practice communication and empathy skills, preparing them to handle difficult conversations with sensitivity and understanding before they meet real patients. Studies show that after training with these virtual patients, students demonstrate more confidence and emotional intelligence, which can improve the quality of their real-life interactions.
The Virtual Dementia Tour (VDT) allows healthcare providers to experience the sensory and cognitive challenges faced by dementia patients. This immersive training helps providers build empathy, fundamentally changing how they approach care for dementia patients. As one participant put it, “Understanding dementia from the inside out has made me more patient and compassionate in my interactions.” This kind of training highlights the unique power of AI in creating experiences that deepen caregivers’ understanding and empathy.
In platforms like Body Interact, virtual patients respond realistically with emotional cues and evolving symptoms, allowing medical students to practice compassionate care in a risk-free setting. This experience helps students bridge the gap between technical skills and the softer, more intuitive skills that are essential for compassionate care. A trainee shared that practicing with virtual patients felt “like having unlimited access to real patients” but with the ability to make and learn from mistakes without consequences, which builds both competence and empathy.
The Road Ahead: Challenges and Promise
A recent review by Mir MM et al. (2023) highlights AI’s growing role, noting advances in error detection, personalized medicine, and adaptive learning that support tailored student development. Yet, challenges remain, especially around bias and the need for more refined, error-free algorithms.
In fact, with these advances come still important questions: how do we guarantee that AI systems are not prejudiced in their teaching? How do we retain the critical human element in medical education while harnessing these incredibly powerful new tools? The answers are as fast moving as the technology itself.
What is obvious is that we are in the middle of a basic transformation of how we prepare health professionals. The question is no longer if AI will transform medical education; it already has! The real challenge now is how to utilize these tools most effectively to develop healthcare providers who are not only skilled but also compassionate, adaptable, and equipped for the demands of future medicine.
The Future is Now: Riding the AI Wave in Healthcare Education
As we look back at how far medical simulation has come, and forward to where it’s heading, one thing becomes clear: this isn’t just another technological trend in healthcare education. We are talking about the fundamental transformation of how we will prepare the next generation of healthcare professionals.
Once limited to imagining symptoms and patient reactions, students now experience lifelike scenarios through advanced simulations, gaining the chance to practice repeatedly and receive instant, objective feedback.
The impact of such a transformation goes much beyond the confines of individual institutions or specialties, AI based simulation is not only enhancing technical skills but also nurturing confident, empathetic, and adaptable professionals prepared for both routine and complex challenges.
The question we need to face is no longer about the adoption of these technologies but rather how best to deploy them in service to both the educator and the learner. Unless otherwise noted, AI simulation is going to make healthcare education more accessible, effective, and responsive to both students’ and patients’ needs.
References
1. Mir MM, Mir GM, Raina NT, et al. Application of Artificial Intelligence in Medical Education: Current Scenario and Future Perspectives. J Adv Med Educ Prof. 2023;11:133-140.
2. Furlan R, et al. A Natural Language Processing-Based Virtual Patient Simulator and Intelligent Tutoring System for the Clinical Diagnostic Process: Simulator Development and Case Study. JMIR Med Inform. 2021 Apr 9;9(4):e24073
3. Ruberto AJ, et al. The future of simulation-based medical education: Adaptive simulation utilizing a deep multitask neural network. AEM education and training, 2021:5(3):e10605
4. Watson RA. Use of a machine learning algorithm to classify expertise: analysis of hand motion patterns during a simulated surgical task. Acad Med. 2014;89(8):1163-1167.
5. Islam G, Kahol K. Application of computer vision algorithm in surgical skill assessment. 7th International Conference on Broadband Communications and Biomedical Applications, Melbourne, VIC, Australia, 2011:108-111
6. Belmar F, et al. Artificial intelligence in laparoscopic simulation: a promising future for large-scale automated evaluations. Surg Endosc. 2023 Jun;37(6):4942-4946
7. Peters AL, Van der Drift G, Van Osch M, et al. The effectiveness of virtual and mixed reality for emergency care training: A systematic review. IEEE Access. 2020;8:58773-58789. https://ieeexplore.ieee.org/document/8993789
8. Mcdougall EM, Shalhoub J, Gautam G, et al. Virtual reality simulation curriculum to teach open emergency surgery. Journal of Surgical Research. 2021;268:1-5. https://www.journalofsurgicalresearch.com/article/S0022-4804(21)00416-9/abstract
9. Williams L. Inside a virtual reality emergency simulator. The MDU Journal. 2023. https://www.themdu.com/for-students/features/this-is-what-its-like-inside-a-virtual-reality-emergency-simulator
10. Kardong-Edgren S, Breitkreuz K, Werb M, et al. Evaluating the usability of virtual simulations for nursing education. ASCILITE Proceedings. 2023;2(1):45-52.
https://publications.ascilite.org/index.php/APUB/article/view/683
11. Beville PK. Virtual Dementia Tour®: A breakthrough technology changing the way we deliver dementia care. NextAvenue. 2022. https://www.nextavenue.org/take-virtual-dementia-tour/
12. BodyInteract. The role of virtual patients in developing communication and empathy skills. BodyInteract Blog. 2023. https://bodyinteract.com/blog/communication-empathy-virtual-patients/
READ ALSO