What if your medical mentor never slept, tracked your every learning move, and knew exactly how to help you improve, instantly? As artificial intelligence (AI) rapidly integrates into medical education, this vision is becoming a reality. But without intuitive dashboards and user-friendly interfaces, even the smartest AI risks becoming just another complicated tool. The key to unlocking AI’s full potential in training future healthcare providers lies not just in the algorithms, but in the design that connects them to the people they aim to support.
The rapid integration of artificial intelligence (AI) into medical education is transforming how students learn, practice, and master essential skills. Yet, for AI tools to deliver meaningful results, they need to be supported by intuitive, well-designed user interfaces (UI) and dashboards that bring clarity to complexity. Dashboards in AI systems function much like the cockpit of an airplane: they provide real-time, actionable data to help students stay on course while managing the inevitable turbulence of medical training.
AI’s relevance in medical education is immense. These systems deliver personalized mentoring by analyzing student performance, diagnosing knowledge gaps, and offering decision support tailored to an individual’s learning path. Imagine an AI-powered dashboard that doesn’t just identify a student’s struggles with patient histories but suggests a precise module or resource to address it—like a mentor who understands every learner uniquely. For this vision to be realized, the interfaces must be intuitive, clear, and built to integrate seamlessly into existing learning modules. Without effective UIs, even the most advanced AI tools risk falling flat.
As noted by Epstein et al. (2020), the aggregation and display of personalized metrics through dashboards can significantly enhance both clinical care and medical training. Epstein et al. highlight that real-time performance analytics, trend visualization, and benchmark comparisons improve learning outcomes by providing residents with clear, actionable insights. Clinical dashboards have been successfully implemented in residency programs, demonstrating increased engagement and more precise self-assessment among medical trainees.
At the Artificial Intelligence Division in Simulation, Education, and Training (AIDSET), we are building on these findings by exploring how these systems can transform learning outcomes by merging technical excellence with human-centered design.
A dashboard isn’t just a tool: it’s a conversation between humans and technology.
When built well, it helps users think better, learn faster, and take the next step with confidence.
The Role of Dashboards and User Interfaces in AI Mentoring Systems
Dashboards centralize data visualization and provide a bridge between complex AI insights and actionable information. For students, they track real-time progress and suggest tailored learning paths. A dashboard might show trends in diagnostic accuracy, flag areas for improvement, or recommend practice modules based on performance. For educators, dashboards reveal class-wide trends and pinpoint individual learning needs, giving them the tools to intervene in a meaningful way. Administrators rely on dashboards to analyze program outcomes and identify opportunities for improvement. Developers can also use them to refine systems over time.
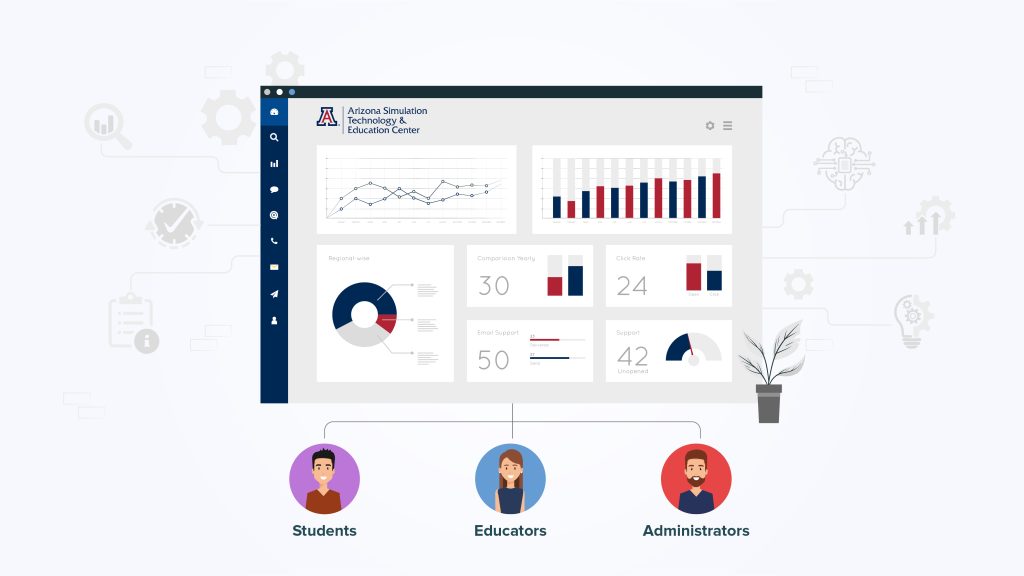
Well-designed dashboards come equipped with key features that support their role as intelligent mentors. Progress tracking highlights student achievements and skill assessments identify their strengths and weaknesses. Personalized learning paths guide students to appropriate content, keeping them on an upward trajectory. Integrating case studies and simulations into dashboards further enhances their practical utility.
For example, an AI-driven dashboard might analyze a student’s performance in diagnosing cardiac conditions, offer targeted feedback, and recommend new case studies for practice, transforming abstract feedback into actionable next steps. The importance of easily accessible and interpretable performance data is emphasized in the literature, as it constitutes critical feedback that facilitates informed self-assessment and learning planning (Yarahuan et al., 2022). Usability studies on dashboards used by pediatric residents reveal high engagement levels when interfaces are designed with automation and intuitive navigation. Yarahuan et al. reported improved efficiency and reduced cognitive overload when dashboards were optimized based on user feedback.
Challenges in Creating Effective UI for AI Mentoring
Designing dashboards that meet the needs of diverse users remains a major challenge. Novice learners require simplified visuals and structured pathways, while advanced students seek in-depth analytics and flexibility. Balancing these needs requires a user-centered approach, ensuring that dashboards are both intuitive and functional. Cognitive overload is another hurdle. Medical education generates vast amounts of data, and without careful design, dashboards risk overwhelming users instead of supporting them. The goal is to distill complexity into clarity, to present data in a way that makes learning actionable, not burdensome.
Personalization plays a pivotal role in effective UI design. Each learner has unique goals and challenges, and dashboards must reflect this diversity. For instance, a student struggling with patient interview techniques might receive recommendations for targeted clinical history modules, while another excelling in diagnostics could be guided toward advanced cases. The dashboard must evolve with the learner, tailoring its insights and recommendations as progress is made. This adaptability is crucial for fostering a culture of reflective practice among medical students (Boscardin et al., 2017).
Balancing simplicity and depth is crucial. Boscardin et al. propose “twelve tips” for dashboard development, emphasizing usability, adaptability, and learner engagement. These insights support the need for user-centered design in medical AI dashboards. Dashboards should facilitate self-regulated learning by providing structured feedback, goal-setting tools, and reflection prompts. This ensures that students continuously refine their skills and learning strategies based on performance metrics.
Another challenge lies in interoperability. AI mentoring systems do not exist in a vacuum; they must integrate seamlessly with electronic health records (EHR), simulation tools, and other educational platforms. Interrupting workflows with siloed tools can alienate users, whereas interconnected systems enhance productivity and ease of use. Ethical considerations, including data privacy and bias mitigation, must also be addressed. Sensitive medical data requires protection under frameworks like HIPAA, and AI systems must be continuously tested to ensure fairness and reliability in their recommendations.
Visualization of AI insights is essential for ensuring that dashboards are accessible to non-technical users. AI algorithms often produce complex outputs, but a well-crafted dashboard translates these results into clear, digestible formats. For example, a comparative graph showing diagnostic performance against AI benchmarks can help students see their standing and offer a clear path for improvement. To make this possible, real-time performance is critical. Dashboards must process data instantaneously to provide timely feedback and ensure that learning remains fluid and uninterrupted.
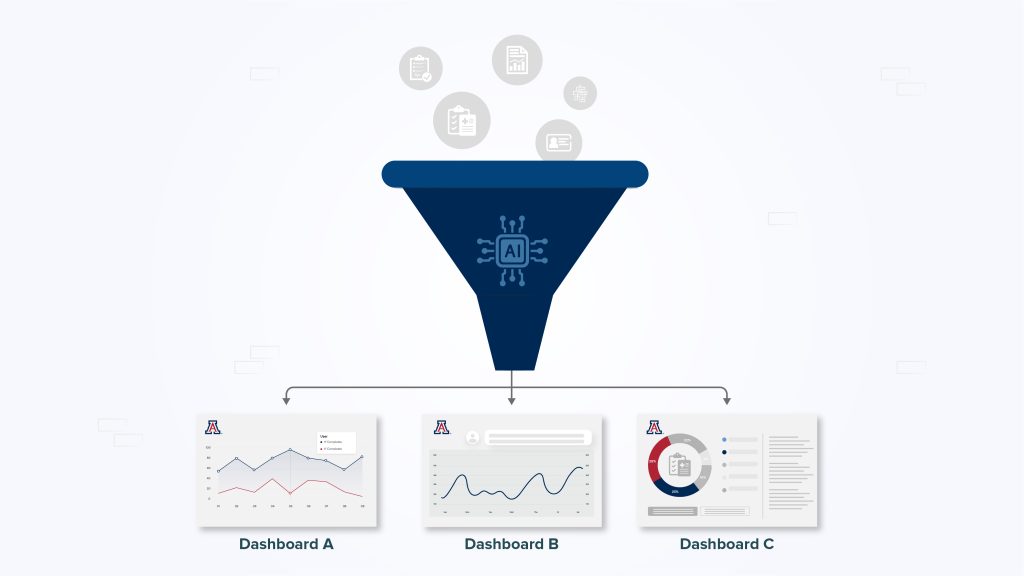
Technical Considerations in Dashboard Design
The technical backbone of an AI dashboard combines both backend and frontend design elements. On the backend, AI models are integrated to analyze large-scale datasets and process real-time insights. Efficient database management ensures that these systems operate smoothly, even as data complexity increases. On the frontend, dashboards must offer engaging, interactive components with responsive design to ensure compatibility across devices. Whether students access them on classroom desktops or hospital tablets, the user experience must remain consistent.
Scalability is another important consideration. As medical education programs expand, dashboards must accommodate increasing numbers of users and growing data volumes without compromising performance. Cloud-based infrastructure provides the flexibility and scalability needed to meet these demands.
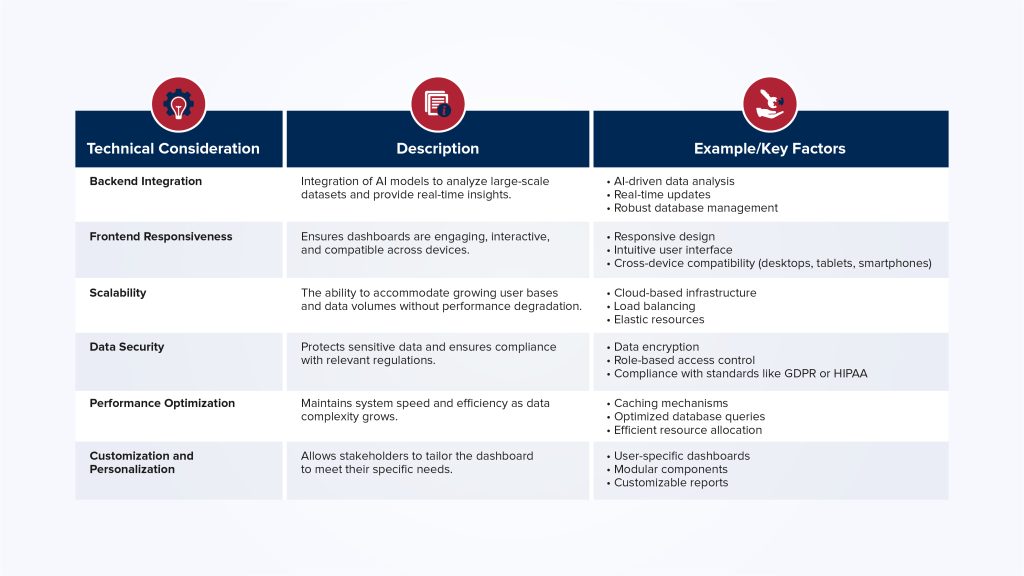
User Engagement and Continuous Improvement
Creating a dashboard is not a one-time effort; it is an iterative process driven by user engagement and feedback. At our lab, we conduct extensive testing with medical students, faculty, and administrators to ensure our tools align with real-world needs. Feedback loops are invaluable, allowing us to refine features, simplify workflows, and address user pain points as they arise. Effective onboarding is equally critical. Tutorials, guides, and training support ensure that students and educators fully understand how to leverage dashboard features.
Case Studies and Best Practices
Theator (Palo Alto, CA) is a surgical UI that provides an excellent example of how AI-driven platforms can enhance medical education through precision insights and real-time feedback. Focused on surgical education, Theator leverages artificial intelligence and computer vision to analyze surgical procedures, providing critical performance insights for both trainees and educators. By capturing and breaking down surgical videos, Theator identifies key moments in procedures, benchmarks them against best practices, and offers detailed feedback that helps improve technical skills and decision-making.
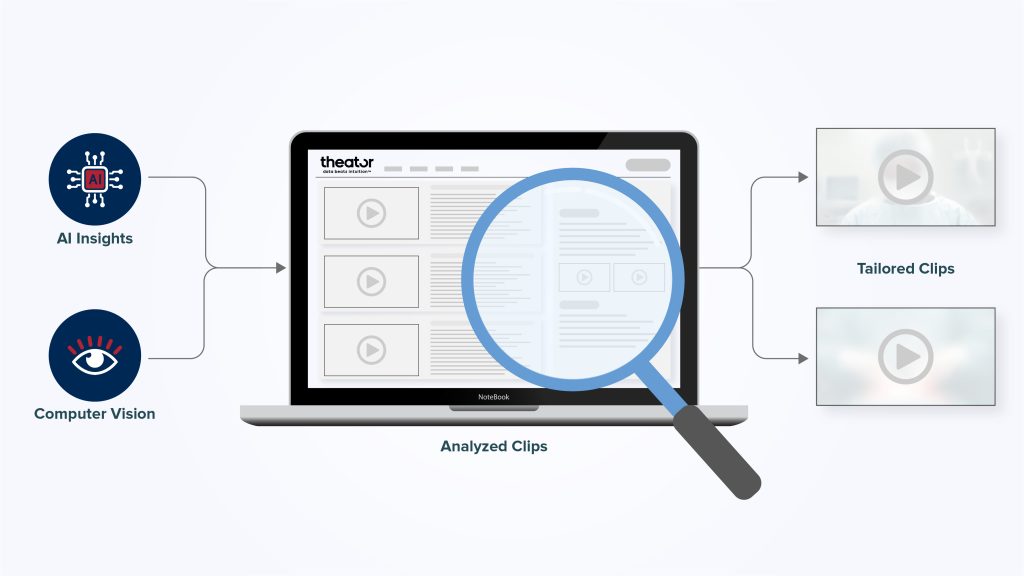
Theator’s dashboard is designed to deliver actionable insights through intuitive visualizations. Surgical trainees can review their procedures, understand where deviations occurred, and receive personalized suggestions for improvement. Educators benefit from aggregated data that highlights common challenges across learners and identifies opportunities for targeted interventions. The platform’s ability to present AI-driven insights in a clear and digestible format makes it particularly effective for teaching complex procedures. This aligns with findings that dashboards should be designed to be accessible, actionable, and clinically relevant to maximize their impact on medical training (Epstein et al., 2020).
One of the major lessons learned from Theator’s implementation is the value of combining real-world case analysis with AI-powered feedback. By integrating seamlessly into existing surgical training programs, Theator enhances traditional methods without disrupting workflows. It also underscores the importance of user-centered design in medical education technology. The dashboard’s ability to simplify intricate surgical data into understandable, actionable insights ensures usability for both novice and advanced learners.
Theator aligns with industry standards for educational and medical UI/UX design, incorporating accessibility principles to ensure its tools are inclusive. Features such as clear data breakdowns, interactive video playback, and consistent navigation create a streamlined experience for users. This approach not only improves individual performance but also supports educators in building more effective, data-driven training programs.
Theator’s success highlights how AI-driven platforms can transform medical education. Its emphasis on real-world application, intuitive design, and measurable outcomes offers a model for future AI mentoring systems.
The Future of AI-Powered Dashboards in Medical Education
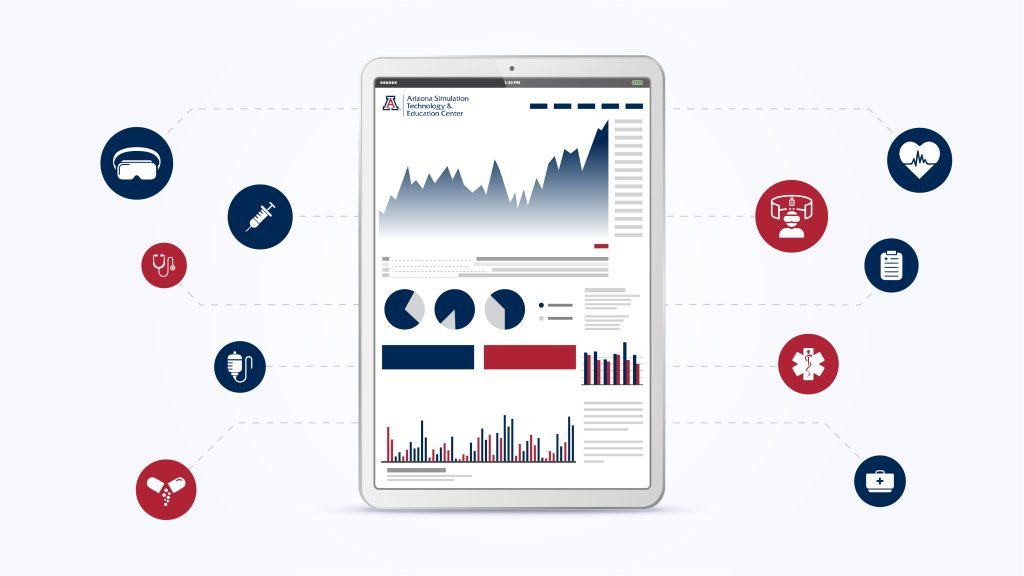
The future of AI mentoring systems holds exciting possibilities. Advances in predictive analytics, natural language processing, and virtual reality will continue to elevate dashboard functionality. For example, VR-based tools could simulate patient interactions in real time while tracking student performance and providing immediate feedback. Similarly, natural language interfaces may allow students to interact with dashboards conversationally, asking questions and receiving tailored insights instantly.
Future AI-powered dashboards will integrate predictive analytics, VR simulations, and natural language processing to enhance medical training and real-time feedback. Chan and Zary (2019) discuss how these technologies personalize medical education by dynamically adapting content to student progress. Predictive analytics allow for early identification of struggling students, enabling timely interventions. Virtual reality simulations enhance procedural training by providing immersive, hands-on practice environments. Natural language processing enables dashboards to function as interactive tutors, responding to student queries and providing real-time guidance. However, institutional and regulatory hurdles remain key challenges to AI adoption in education. Addressing these barriers in dashboard design ensures compliance with data privacy standards and promotes broader implementation in academic programs.
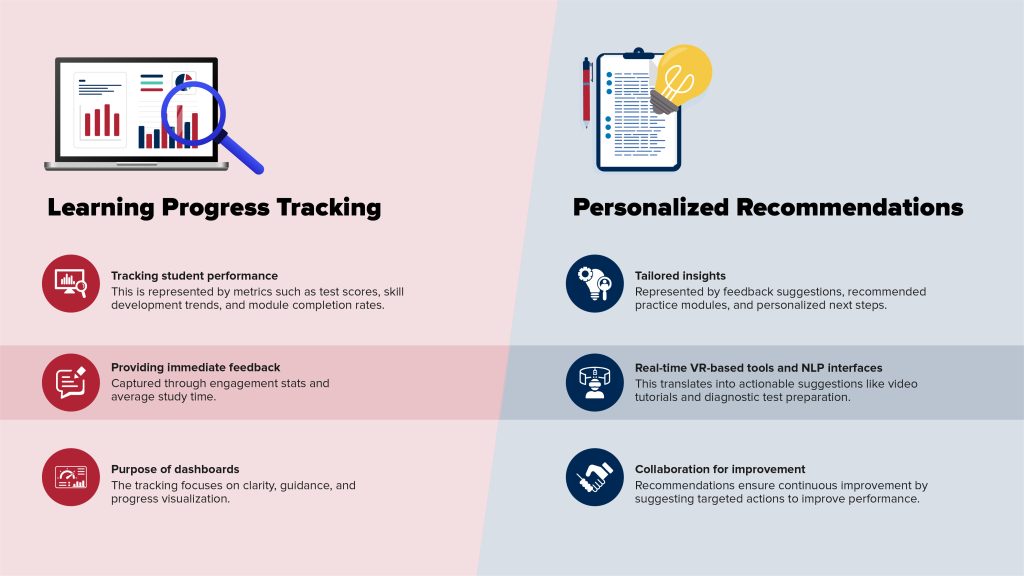
As dashboards evolve, their purpose remains unchanged: to provide clarity, guidance, and actionable insights that help medical students become better learners and, ultimately, better doctors. Achieving this vision requires collaboration. Educators, developers, and medical professionals must work together to overcome challenges, refine tools, and ensure that AI systems empower learners at every stage of their education. At our lab, we believe that well-designed AI tools are more than technological advancements, they are instruments that shape the future of medical education.
References
Epstein et al. “Smarter Screen Time: Integrating Clinical Dashboards Into Graduate
Medical Education” Journal of Graduate Medical Education (2020) doi:10.4300/jgme-d-19-00584.1
Yarahuan et al. “Design, Usability, and Acceptability of a Needs-Based, Automated
Dashboard to Provide Individualized Patient-Care Data to Pediatric Residents” Applied
Clinical Informatics (2022) doi:10.1055/s-0042-1744388
Boscardin et al. “Twelve tips to promote successful development of a learner performance
dashboard within a medical education program” Medical Teacher (2017)
doi:10.1080/0142159x.2017.1396306
Chan and Zary “Applications and Challenges of Implementing Artificial Intelligence in
Medical Education: Integrative Review” JMIR Medical Education (2019) doi:10.2196/13930
Theator | The Surgical Intelligence Company. (n.d.). Theator | the Surgical Intelligence Company. https://theator.io/
READ ALSO